Revolutionizing Robot Control: A Game-Changing Machine Learning Technique
MIT and Stanford University researchers have made groundbreaking advancements in the field of robotics by developing a novel machine-learning technique. This technique can potentially revolutionize the control of robots, including drones and autonomous vehicles, in dynamic environments with rapidly changing conditions.
The researchers integrated principles from control theory into the machine-learning process, leading to the creation of more efficient and effective controllers. The objective was to learn intrinsic structures within the system dynamics that could be leveraged to design superior stabilizing controllers.
What sets this approach apart is the incorporation of control-oriented structures into the model learning process. Unlike traditional methods, which require separate steps to derive or learn controllers, this new approach immediately extracts an effective controller from the learned model.
The inspiration for this method came from how roboticists use physics to derive simpler robot models. These manually derived models capture essential structural relationships based on the system’s physics. However, in complex systems, manual modeling becomes impractical, leading researchers to use machine learning to fit a model to the data.
#MIT and #Stanford researchers developed a #ML method for better robot control in changing conditions. The approach learns control-oriented structures, effectively controlling complex dynamics and outpacing traditional models.
#AI #RoboticsControlhttps://t.co/CQ8LfGm00R
— SwissCognitive, World-Leading AI Network (@SwissCognitive) July 27, 2023
The MIT and Stanford teams incorporated control-oriented structures during the machine learning process to address this limitation. This approach combines the physics-inspired method with data-driven learning, resulting in controllers extracted directly from the learned dynamics model.
During testing, the new controller closely followed desired trajectories and outperformed various baseline methods. Surprisingly, the controller derived from the learned model almost matched the performance of a ground-truth controller built using exact system dynamics.
Another impressive aspect of the technique is its data efficiency, achieving outstanding performance with minimal data points. This characteristic makes it particularly promising for real-world applications where robots or drones must adapt quickly to rapidly changing conditions.
Moreover, the approach’s generality allows it to be applied to various dynamical systems, such as robotic arms and free-flying spacecraft operating in low-gravity environments.
Looking ahead, the researchers aim to develop more interpretable models that will enable them to identify specific information about a dynamic system. This could lead to even better-performing controllers, further advancing the field of nonlinear feedback control.
Experts in the field have praised the contributions of this research, highlighting the integration of control-oriented structures as an inductive bias in the learning process. This conceptual innovation has led to a highly efficient learning process, resulting in dynamic models with intrinsic structures conducive to effective, stable, and robust control.
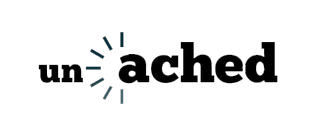